Machine Learning In a Nutshell: A Comprehensive Guide for Executive Leaders

In today's rapidly evolving technological landscape, machine learning (ML) has emerged as a transformative force that is reshaping industries and organizations across the globe. For executive leaders, it is imperative to have a clear understanding of ML's capabilities and applications to leverage its full potential and drive innovation within their organizations.
4.1 out of 5
Language | : | English |
File size | : | 1383 KB |
Text-to-Speech | : | Enabled |
Screen Reader | : | Supported |
Enhanced typesetting | : | Enabled |
Print length | : | 81 pages |
Lending | : | Enabled |
This comprehensive guide provides an overview of ML for executive leaders, covering key concepts, applications, and strategies for effective implementation. By gaining a deeper understanding of this technology, leaders can empower their organizations to make informed decisions, optimize operations, and gain a competitive edge in the digital age.
Key Concepts of Machine Learning
ML is a subfield of artificial intelligence (AI) that focuses on developing algorithms that can learn from data without explicit programming. These algorithms can identify patterns, make predictions, and adapt to changing circumstances, enabling machines to perform tasks that traditionally require human intelligence.
The key concepts of ML include:
- Data: ML algorithms require large amounts of data to learn from. This data can be structured, unstructured, or a combination of both.
- Algorithms: ML algorithms are statistical models that learn from data and make predictions. Common ML algorithms include linear regression, decision trees, and neural networks.
- Training: The process of building an ML model involves training it on a dataset. During training, the model learns to identify patterns and relationships within the data.
- Evaluation: Once an ML model is trained, it needs to be evaluated to assess its accuracy and performance. Evaluation metrics vary depending on the specific application.
- Deployment: Once an ML model is evaluated and meets performance expectations, it can be deployed to make predictions on new data.
Applications of Machine Learning
ML has a wide range of applications across various industries and domains. Some common applications include:
- Predictive analytics: ML algorithms can predict future events or outcomes based on historical data. This is used in areas such as demand forecasting, risk assessment, and fraud detection.
- Image and speech recognition: ML algorithms can be used to identify objects, images, and spoken words. This is applied in areas such as facial recognition, medical imaging, and customer service chatbots.
- Natural language processing (NLP): ML algorithms can understand and process human language. This is used in areas such as machine translation, text summarization, and spam filtering.
- Recommendation systems: ML algorithms can be used to recommend products, movies, or other items to users based on their preferences and historical behavior. This is used in areas such as e-commerce, streaming services, and social media.
- Automation: ML algorithms can automate tasks that are repetitive or require human judgment. This is used in areas such as customer support, data entry, and manufacturing.
Strategies for Leveraging Machine Learning
To effectively leverage ML within organizations, executive leaders should consider the following strategies:
- Identify clear business objectives: Before implementing ML, it is important to identify specific business objectives that ML can help achieve. This will ensure that ML projects are aligned with overall organizational goals.
- Invest in data infrastructure: ML algorithms require large amounts of data to learn from. Organizations need to invest in data collection, storage, and management infrastructure to support ML initiatives.
- Build a team of ML experts: Building and deploying ML models requires specialized skills. Organizations should invest in hiring and training ML engineers, data scientists, and other technical experts.
- Foster a culture of data-driven decision-making: ML can help organizations make data-driven decisions by providing insights and predictions. Leaders should promote a culture where data is valued and used to inform strategic decisions.
- Monitor and evaluate ML projects: ML models need to be monitored and evaluated regularly to ensure they are performing as expected. Organizations should establish metrics and KPIs to track the success of ML projects.
Machine learning is a powerful technology that has the potential to transform organizations and drive innovation across industries. By understanding the key concepts, applications, and strategies for leveraging ML, executive leaders can empower their organizations to make informed decisions, optimize operations, and gain a competitive edge in the digital age.
Embracing ML requires a commitment to data, analytics, and a culture of continuous learning. By investing in the right people, processes, and infrastructure, organizations can unlock the full potential of ML and achieve lasting success in today's data-driven business environment.
4.1 out of 5
Language | : | English |
File size | : | 1383 KB |
Text-to-Speech | : | Enabled |
Screen Reader | : | Supported |
Enhanced typesetting | : | Enabled |
Print length | : | 81 pages |
Lending | : | Enabled |
Do you want to contribute by writing guest posts on this blog?
Please contact us and send us a resume of previous articles that you have written.
Book
Novel
Text
Genre
Magazine
Newspaper
Paragraph
Sentence
Bookmark
Shelf
Foreword
Preface
Footnote
Manuscript
Scroll
Tome
Bestseller
Classics
Library card
Narrative
Memoir
Reference
Encyclopedia
Thesaurus
Resolution
Librarian
Stacks
Archives
Study
Research
Scholarly
Reading Room
Rare Books
Special Collections
Literacy
Study Group
Thesis
Dissertation
Book Club
Textbooks
Karen M Paget
Amilia Quinn
Kim Cooper
Julia Kelly
Abigail Kessler
Joan Aiken
Michele Stolfa
Marian White
Scott Green
Susan Sloan
1st Edition Kindle Edition
Dennis Wang
Angela Bennett
Tony Hawks
J T Ward
Katherine Spielmann
Herbert H Harwood
Abby Jane Morrell
Tim Alan Garrison
Rayna Gillman
Light bulbAdvertise smarter! Our strategic ad space ensures maximum exposure. Reserve your spot today!
- Henry Wadsworth LongfellowFollow ·7k
- Jerome PowellFollow ·19.2k
- Davion PowellFollow ·18.6k
- Bryan GrayFollow ·17.7k
- Cooper BellFollow ·12.8k
- Frank MitchellFollow ·7.5k
- Anton ChekhovFollow ·17.5k
- Arthur MasonFollow ·17.7k
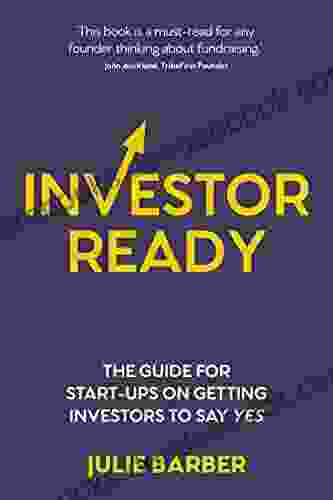

The Complete Guide for Startups: How to Get Investors to...
Are you a startup...
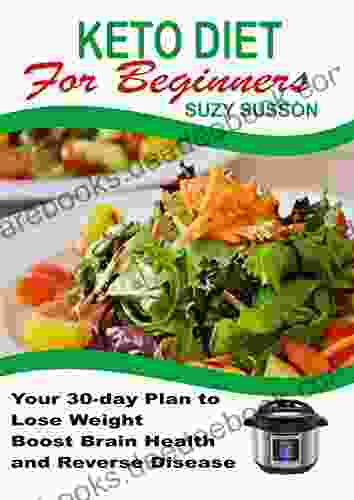

Your 30 Day Plan To Lose Weight, Boost Brain Health And...
Are you tired of feeling tired, overweight,...
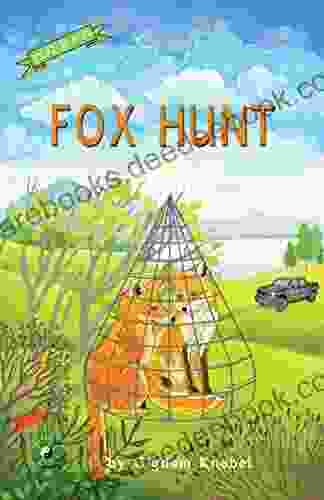

Fox Hunt: (Dyslexie Font) Decodable Chapter (The Kent S...
What is Dyslexia? Dyslexia is a...
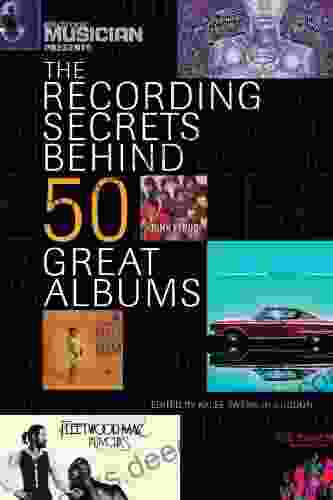

Electronic Musician Presents: The Recording Secrets...
By [Author's Name] In the world of music,...
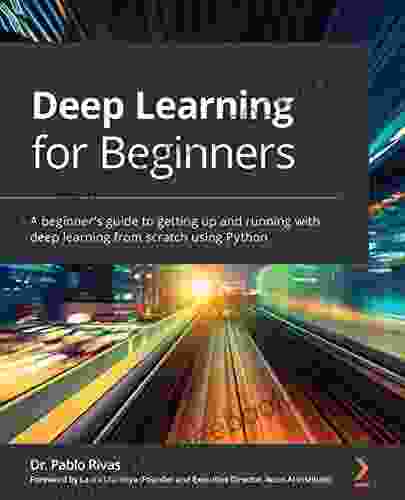

A Comprehensive Guide to Deep Learning for Beginners
Deep learning is a subfield...
4.1 out of 5
Language | : | English |
File size | : | 1383 KB |
Text-to-Speech | : | Enabled |
Screen Reader | : | Supported |
Enhanced typesetting | : | Enabled |
Print length | : | 81 pages |
Lending | : | Enabled |