Explainable Artificial Intelligence For Cyber Security: A Comprehensive Overview

In the ever-evolving landscape of cyber security, the integration of Artificial Intelligence (AI) has revolutionized the detection and response capabilities of security teams. However, the complexity and opacity of many AI models have hindered their widespread adoption, raising concerns about interpretability and accountability in decision-making.
4.9 out of 5
Language | : | English |
File size | : | 25843 KB |
Text-to-Speech | : | Enabled |
Enhanced typesetting | : | Enabled |
Print length | : | 481 pages |
Screen Reader | : | Supported |
This article provides a comprehensive overview of Explainable Artificial Intelligence (XAI),a subset of AI that focuses on making the models and their decisions interpretable and understandable to human users. We will explore the significance of XAI in cyber security, examine different XAI techniques, and discuss the benefits and challenges of using XAI for enhanced cyber threat detection and response.
Significance of XAI in Cyber Security
In the context of cyber security, XAI plays a crucial role in addressing the following challenges:
- Enhancing Trust and Accountability: XAI provides transparency into the decision-making process of AI models, building trust and accountability among stakeholders.
- Facilitating Human Oversight: Interpretable models enable security analysts to validate the decisions made by AI systems, ensuring that the actions taken are aligned with organizational policies and legal requirements.
- Improving Incident Response: By understanding the rationale behind AI-driven alerts, analysts can prioritize and respond to incidents more effectively, reducing the risk of false positives and missed threats.
- Supporting Legal and Regulatory Compliance: XAI helps organizations demonstrate compliance with regulations that require transparency and accountability in AI usage, such as the European Union's General Data Protection Regulation (GDPR).
XAI Techniques for Cyber Security
Numerous XAI techniques can be applied to enhance the interpretability of AI models in cyber security. Some notable approaches include:
- Model Agnostic Explainability: Techniques like LIME (Local Interpretable Model-Agnostic Explanations) and SHAP (SHapley Additive Explanations) can be used to explain the predictions of complex black-box models by approximating them with simpler, interpretable models.
- Feature Importance Analysis: By quantifying the contribution of each feature to the model's predictions, techniques like Gini importance and permutation importance provide insights into the factors driving the model's decisions.
- Counterfactual Analysis: This technique explores alternative scenarios to help understand how changes in input features would affect the model's predictions, providing a deeper understanding of the model's behavior.
- Visual Explanations: Visualization techniques such as heatmaps, decision trees, and graphs can help visualize the model's predictions and make them more comprehensible to users.
Benefits of Using XAI in Cyber Security
The integration of XAI in cyber security offers significant benefits, including:
- Enhanced Detection and Response Capabilities: XAI empowers security teams with a deeper understanding of AI-driven alerts, leading to faster and more accurate incident response.
- Improved Risk Management: By identifying the factors that contribute to cyber threats, XAI enables organizations to prioritize risk mitigation strategies.
- Increased Stakeholder Confidence: Interpretable models build trust among stakeholders, fostering collaboration and supporting the adoption of AI in cyber security operations.
- Accelerated Research and Development: XAI techniques provide valuable insights into AI model behavior, facilitating research and development for more robust and trustworthy AI systems.
Challenges of Implementing XAI in Cyber Security
Despite the numerous benefits, there are several challenges associated with implementing XAI in cyber security:
- Increased Computational Cost: XAI techniques can be computationally expensive, especially for large and complex models, posing resource utilization challenges.
- Limited Interpretability: While XAI aims to enhance interpretability, it does not guarantee that all models can be fully understood by human users.
- Trade-offs with Performance: Implementing XAI techniques may introduce a trade-off between model interpretability and performance, requiring careful balancing.
- Lack of Standardization: The field of XAI is still evolving, and there is a lack of standardized methodologies and reporting formats for explaining AI decisions.
Explainable Artificial Intelligence (XAI) holds immense promise for enhancing cyber security operations. By providing transparency and interpretability into AI models, XAI empowers security teams to make more informed decisions, improve incident response, and strengthen risk management. However, it is crucial to address the challenges associated with implementing XAI and carefully consider the trade-offs between interpretability and performance.
As XAI techniques continue to evolve and mature, we can expect to see increased adoption of AI in cyber security, leading to more robust, trustworthy, and human-centered AI systems that protect our digital infrastructure.
4.9 out of 5
Language | : | English |
File size | : | 25843 KB |
Text-to-Speech | : | Enabled |
Enhanced typesetting | : | Enabled |
Print length | : | 481 pages |
Screen Reader | : | Supported |
Do you want to contribute by writing guest posts on this blog?
Please contact us and send us a resume of previous articles that you have written.
Book
Text
Story
Reader
Library
Paperback
E-book
Magazine
Newspaper
Paragraph
Sentence
Bibliography
Synopsis
Annotation
Footnote
Manuscript
Scroll
Codex
Tome
Biography
Memoir
Thesaurus
Narrator
Resolution
Librarian
Catalog
Card Catalog
Stacks
Archives
Study
Scholarly
Lending
Reserve
Journals
Rare Books
Special Collections
Study Group
Thesis
Dissertation
Reading List
J Rock
Meara Platt
Phyllis Alsdurf
Erica S Perl
Elizabeth Atkinson
Steven Bavaria
Kevin Dockery
L S O Dea
Alexandra Paucescu
Donna Quesada
David Antram
Gordon Yu
Oskar Reponen
Nadine Holdsworth
Kathryn M Price
Steve Bickerstaff
Scott Conroy
Thomas Rudolph
Ari Honarvar
Chen Kuczynski
Light bulbAdvertise smarter! Our strategic ad space ensures maximum exposure. Reserve your spot today!
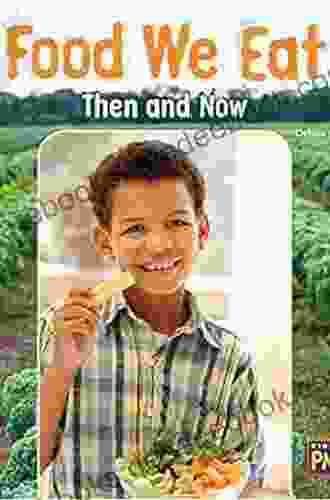

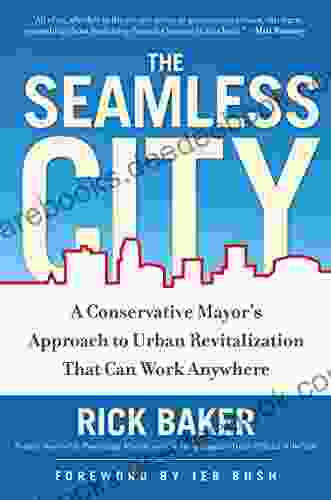

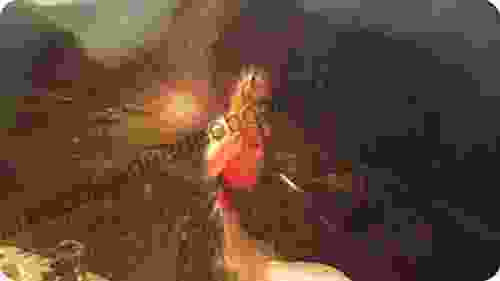

- Tennessee WilliamsFollow ·19.2k
- Stanley BellFollow ·11.1k
- Ethan MitchellFollow ·15.4k
- Brett SimmonsFollow ·10.9k
- Justin BellFollow ·15.2k
- Italo CalvinoFollow ·9.2k
- Mason PowellFollow ·10.4k
- Allan JamesFollow ·13.1k
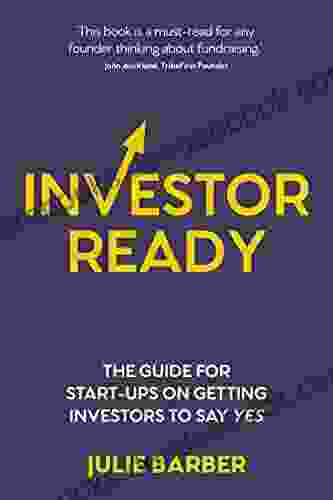

The Complete Guide for Startups: How to Get Investors to...
Are you a startup...
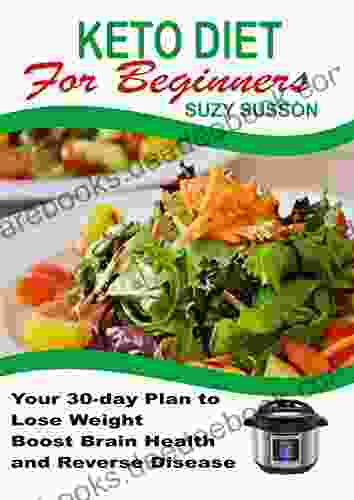

Your 30 Day Plan To Lose Weight, Boost Brain Health And...
Are you tired of feeling tired, overweight,...
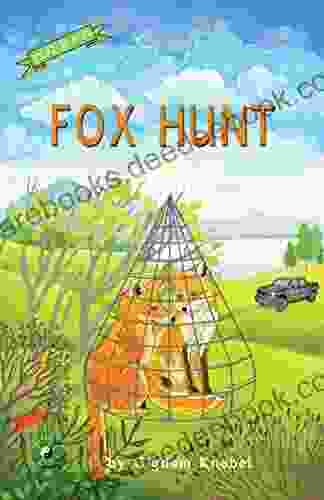

Fox Hunt: (Dyslexie Font) Decodable Chapter (The Kent S...
What is Dyslexia? Dyslexia is a...
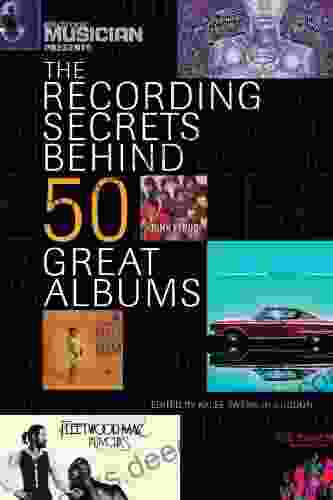

Electronic Musician Presents: The Recording Secrets...
By [Author's Name] In the world of music,...
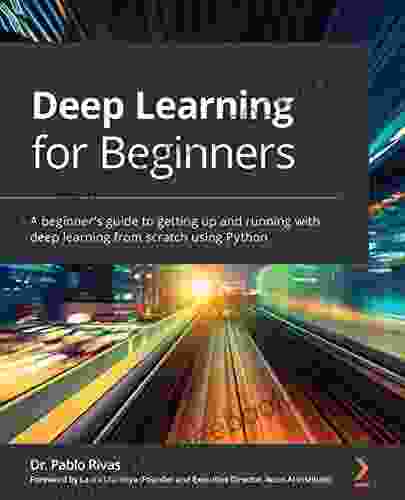

A Comprehensive Guide to Deep Learning for Beginners
Deep learning is a subfield...
4.9 out of 5
Language | : | English |
File size | : | 25843 KB |
Text-to-Speech | : | Enabled |
Enhanced typesetting | : | Enabled |
Print length | : | 481 pages |
Screen Reader | : | Supported |